
Methodology
Using GIS software, spatial aggregation and a host of Python regression libraries, our team created a robust modeling framework to assess the relationship between community gardens and other elements of social infrastructure on mental health outcomes at the census tract level.
Geographically Weighted Regression Model
Central to our analysis is the mgwr package from the Python Spatial Analysis Library (PySal), which performs Geographically Weighted Regressions to account spatial nonstationarity within a dataset.
GWR uses an N x N spatial weighting matrix to create localized datasets and performs regression analysis on each area using only nearby observations, thus accounting for local relationships that may not be captured at the global level.
Target Variable
Percentage of Respondents aged ≥18 years who report 14 or more days during the past 30 days during which their mental health was not good.
Explanatory Variables
Population-normalized community garden counts and social infrastructure counts (from PLUTO building class data) alongside several other relevant socioeconomic, demographic, and community variables.
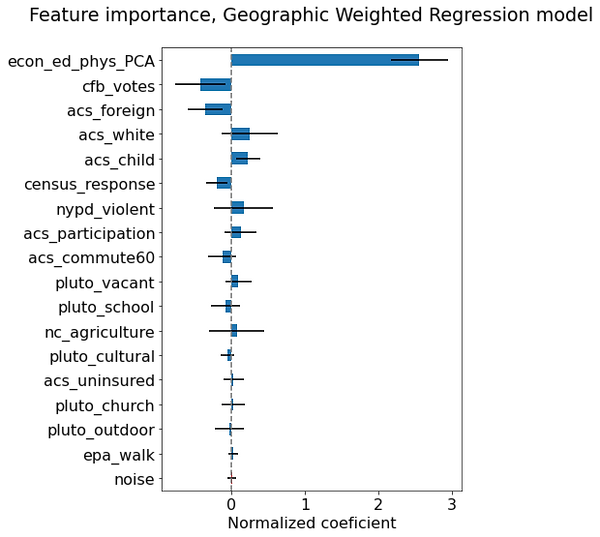
Takeaways
-
Income, Education level, and physical health explain majority of variance across census tracts
​
-
Mental health outcomes are significantly spatially clustered, limiting the explanatory power of global regression model
​
-
Conditional Maps of Local Moran’s I clustering demonstrates a compounding effect of gardens on income level, correlating with clusters of better mental health among high income groups while clusters of worse mental health are present for low income observations.
​
-
Localized impact of social infrastructure and community gardens on mental health varies dramatically across New York City, with our model producing strong positive and negative coefficients in certain areas.